Machine learning is growing fast; the market is expected to increase 36% between 2024 and 2030! That’s huge, and it’s why so many companies and professionals like you are looking into machine learning right now. But here’s the challenge: Which platform should you use? AWS SageMaker vs Azure ML? It’s not an easy decision, but don’t worry, that’s exactly what I will break down.
If you’re a beginner, Azure ML still leads in simplicity. It offers a visual drag-and-drop interface called Designer and a powerful AutoML feature that automatically builds your models. The experience feels more guided, and everything is wrapped in a clean, unified portal called Azure AI Studio, which also connects to other AI tools like OpenAI. Super handy if you’re new to machine learning.
But AWS SageMaker has made huge strides, too. Tools like SageMaker Canvas (a no-code interface) and Autopilot (for automatic model building) make it easier to start without writing code. And with the new Unified SageMaker Studio, AWS has finally put all its ML tools, data prep, notebooks, training, and deployment in one place. Add in Amazon Q, their AI coding assistant, and it’s a much smoother ride than it used to be.
So who wins? Azure ML is still the easiest for non-coders or teams looking for a low-code experience. But if you want deeper customization, especially if you’re already in the AWS ecosystem, SageMaker in 2025 is way more beginner-friendly than before, while still giving pros the power they need.
Which One Handles AI Workloads Better: AWS SageMaker vs Azure ML?
Both platforms are powerful, but the best choice depends on the type of project you’re working on.
If you’re dealing with massive datasets and complex AI models, AWS SageMaker is a beast. It’s designed for high scalability and can train models on petabyte-scale datasets using distributed GPUs. It also integrates seamlessly with AWS services like S3 and EC2, making data processing more efficient.
But if you need hybrid deployments, meaning you want to run AI models both on the cloud and on-premises, Azure ML is the smarter choice. It has high-performance compute clusters, supports GPUs and FPGAs, and is great for companies needing deployment flexibility.
Bottom line? If you’re working on huge, cloud-native AI projects, SageMaker is the way to go. But if you need a hybrid or on-prem deployment, Azure ML might be a better fit.
Build smarter, faster AI apps with our machine learning LATAM Team.
Will It Work With Your Current Tools?
If you’re already using AWS, then SageMaker is the obvious choice. It works seamlessly with S3 for storage, Lambda for event-driven tasks, Redshift for data warehousing, and it has built-in MLOps features that make it easy to automate and monitor your AI models.
On the other hand, if your company is deeply embedded in the Microsoft ecosystem, then Azure ML is the better choice. It integrates well with Azure Data Lake, Databricks for big data processing, Power BI, Synapse Analytics, and Microsoft’s Cognitive Services.
So, this decision is pretty straightforward: SageMaker for AWS users, Azure ML for Microsoft users.
AWS SageMaker vs Azure ML Cost Comparison
Let’s talk about money. Both SageMaker and Azure ML use a pay-as-you-go pricing model, meaning you pay based on how much you use. But there are some key differences.
AWS SageMaker has a Spot Training feature, which lets you run training jobs on unused AWS computing power at a lower cost. That’s a huge advantage if you’re working on a large-scale project and want to optimize costs.
Azure ML, on the other hand, has a more structured pricing model. It starts with a free tier and then offers clear pricing tiers—from $9.99 per month for basic use to $9,999.98 for enterprise-grade AI workloads. If you like knowing exactly how much you’ll pay, Azure ML is the safer bet.
If you want more cost flexibility, SageMaker is a better option. But if you prefer a predictable pricing structure, go with Azure ML.
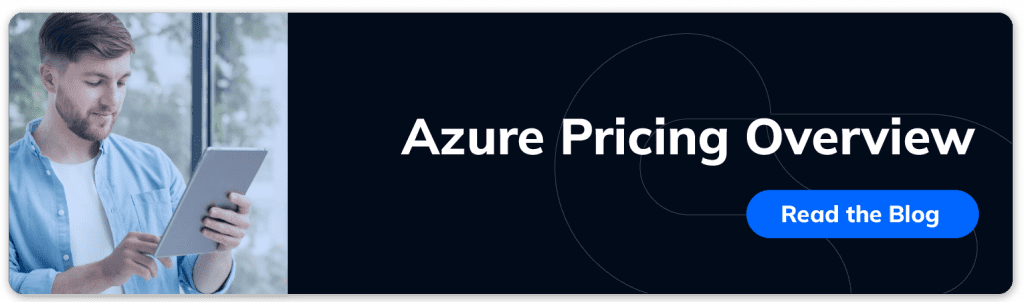
Which One Keeps Your Data Safer?
Security is a big deal, especially if you’re working with sensitive data.
AWS SageMaker uses AWS Identity and Access Management (IAM) to control access, and it has Virtual Private Cloud (VPC) [agregar los iconos de estas dos tecnologías] isolation for added security. It’s a great option if you’re already working within AWS, but since it’s designed primarily for cloud-native environments, it’s not as flexible if you need on-prem security.
Azure ML, on the other hand, offers hybrid security options. It uses Azure Active Directory for authentication, and Confidential Computing adds an extra layer of protection for sensitive AI workloads. Plus, Azure ML has more compliance certifications, making it ideal for industries that have strict security regulations.
So, if you’re already on AWS, SageMaker’s security will feel familiar. But if you need hybrid or on-prem security, Azure ML is the safer bet.
If you’re an AWS-native team, working on large, cloud-based AI projects, or need MLOps and cost optimization, AWS SageMaker is your best choice.
If you need hybrid or on-prem deployments, a user-friendly interface, and a clear pricing model, Azure ML is the way to go.
And here’s something interesting: Some companies actually use both, leveraging SageMaker for model training and Azure ML for deployment. So, if your company is open to a hybrid approach, that might be worth considering!
Optimize your Azure ML setup with our certified Azure specialists.
FAQs
SageMaker is tightly integrated with the AWS ecosystem and offers features like SageMaker Studio, built-in algorithms, and managed Jupyter notebooks.
Azure ML integrates well with Microsoft tools like Power BI, Azure DevOps, and supports low-code/drag-and-drop interfaces through Designer.
SageMaker is often preferred for advanced control and automation, while Azure ML offers a more enterprise-friendly interface and hybrid deployment options.
AWS SageMaker integrates seamlessly with AWS tools like S3, Lambda, CloudWatch, and CodePipeline, making it ideal for teams already using AWS.
Azure ML works well with Azure Data Lake, Power BI, Azure DevOps, and allows integration with GitHub, VS Code, and MLflow for experiment tracking.
Choose SageMaker if you need tight AWS service integration, or go with Azure ML for cross-service Microsoft compatibility and easier MLOps setup.
Azure ML is generally considered more beginner-friendly, thanks to its intuitive UI, drag-and-drop Designer, and strong documentation aimed at enterprises.
AWS SageMaker, while extremely powerful, has a steeper learning curve and is better suited for developers familiar with AWS services.